In the vast and intricate world of bioinformatics, unsupervised learning serves as a powerful tool in uncovering hidden connections and patterns within complex biological data. One particularly fascinating application of this technique is the discovery of links between disparate pieces of genetic information, shedding light on the inner workings of life itself. Join us as we delve into the realm of unsupervised learning and its role in unlocking the secrets of bioinformatics.
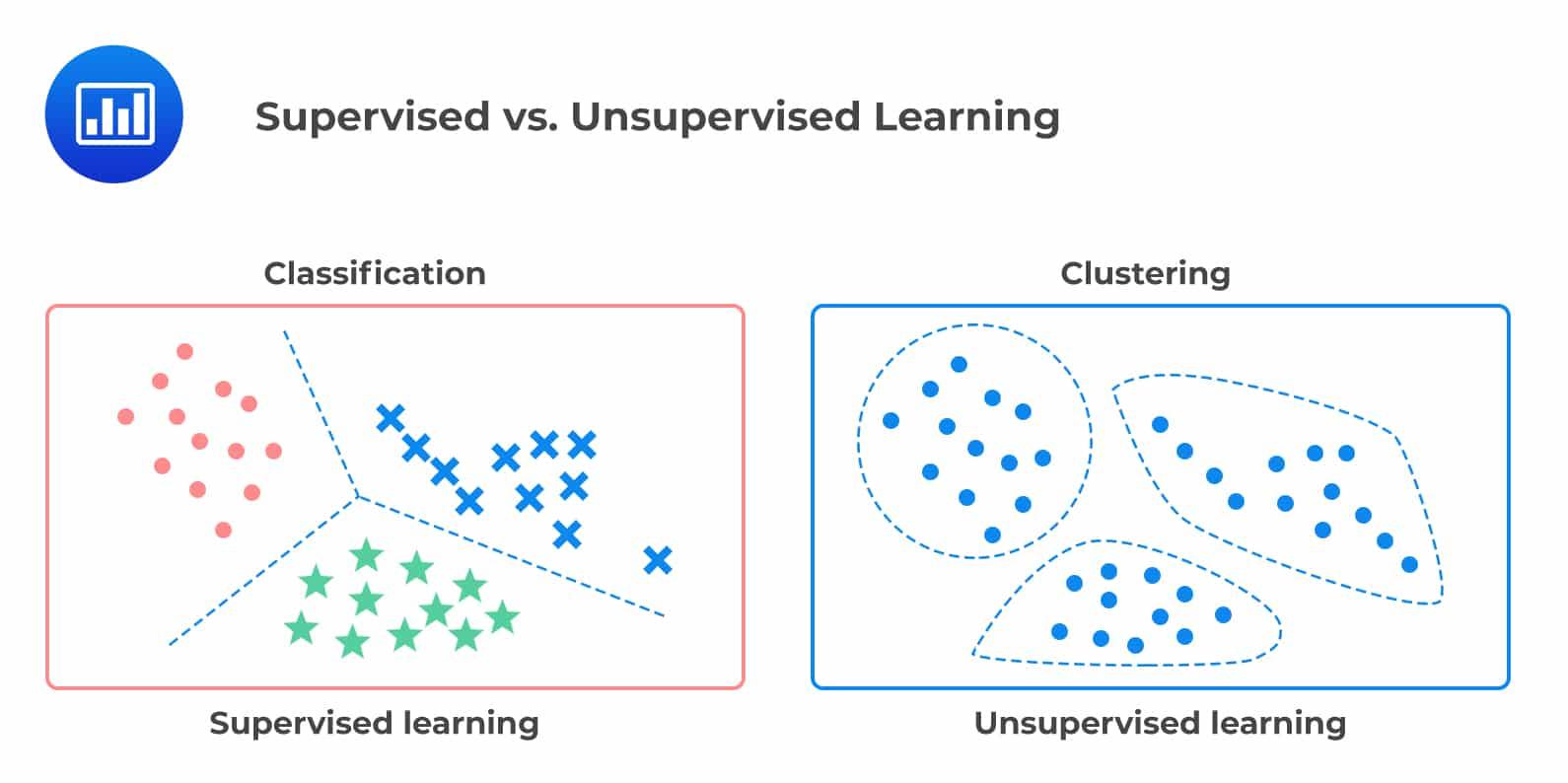
– Understanding Unsupervised Learning in Bioinformatics
When working with unsupervised learning in bioinformatics, one important aspect to consider is the use of modern web compression technologies for static content like PNGs, JPEGs, and SVGs. By implementing practices such as Static Content Compression, you can optimize the delivery of images on your bioinformatics platform, improving user experience and overall performance.
Exploring the sitemap of a bioinformatics website can reveal valuable insights and connections for link discovery. For instance, navigating through sections like Lifecode blog and Rails Directory can help users discover related content on Ruby documentation, Rails practices, and more. Additionally, understanding the Figure-Ground Relationship in the context of web code practice can enhance the long-term memory network of users interacting with your bioinformatics platform.
– The Importance of Link Discovery for Data Integration in Bioinformatics

Our newsletter, with a growing community of over 10,000 subscribers, is a weekly fresh journal that dives deep into the world of AI and automation. In the next issue, we explore the importance of link discovery for data integration in bioinformatics.
Unsupervised learning plays a crucial role in this process, as it allows for the discovery of hidden patterns and relationships within vast amounts of biological data. By leveraging cutting-edge algorithms and techniques, researchers can uncover valuable insights that can lead to breakthroughs in genomics, proteomics, and personalized medicine.
– Challenges and Recommendations for Applying Unsupervised Learning in Bioinformatics
Unsupervised Learning and Link Discovery in Bioinformatics
The application of unsupervised learning techniques in bioinformatics poses both challenges and opportunities. One of the main challenges is the complexity and high dimensionality of biological data, which can make it difficult to extract meaningful patterns without labeled data. Additionally, the noisy and heterogeneous nature of biological data can lead to a higher risk of false positives and overfitting when using unsupervised learning algorithms.
One recommendation for overcoming these challenges is to incorporate domain knowledge and prior biological information into the unsupervised learning process. This can help guide the discovery of relevant biological patterns and relationships in the data. Another recommendation is to use ensemble learning techniques, such as combining multiple unsupervised algorithms or using a combination of unsupervised and supervised learning, to improve the robustness and accuracy of the results. By leveraging these strategies, researchers can enhance the effectiveness of unsupervised learning in bioinformatics and uncover novel insights from biological data.
Insights and Conclusions
unsupervised learning holds great potential for revolutionizing the field of bioinformatics, particularly in the area of link discovery. By allowing algorithms to uncover hidden patterns and connections in vast amounts of data without the need for human supervision, we can unlock new insights into the complex relationships that govern biological systems. As technology continues to advance, we can expect even more exciting developments in this rapidly evolving field. Stay tuned for the latest updates and breakthroughs in unsupervised learning in bioinformatics.